Unlocking Potential: The Importance of Annotated Image Datasets for AI
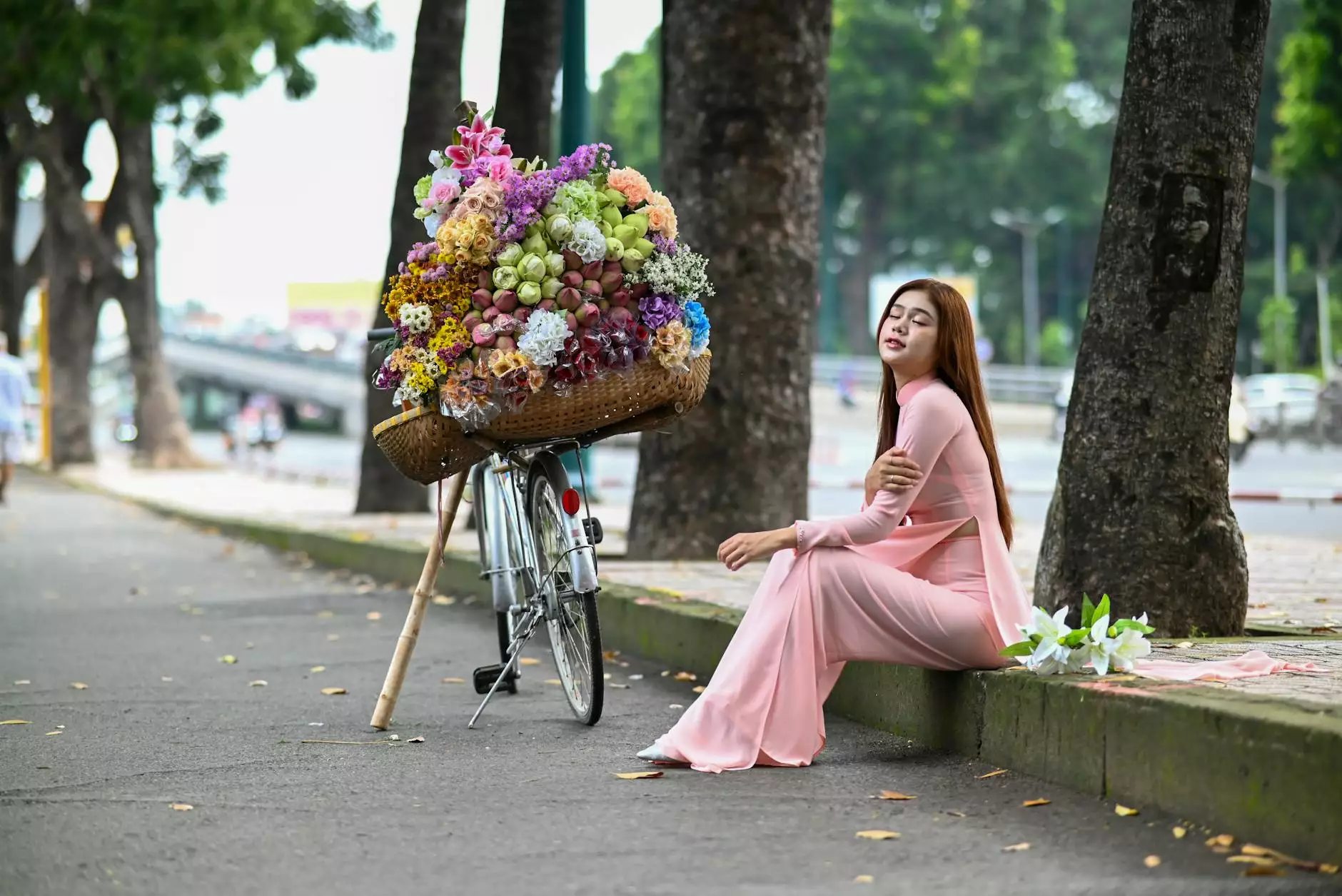
In the fast-evolving landscape of artificial intelligence, the need for high-quality data cannot be overstated. One of the most crucial components for training machine learning models is the use of annotated image datasets. These datasets form the backbone of numerous AI applications, ranging from computer vision to autonomous vehicles. In this article, we will explore the significance of annotated image datasets, the advantages of using advanced data annotation tools and platforms, and how businesses like KeyLabs.ai are leading the charge in this field.
What is an Annotated Image Dataset?
An annotated image dataset is a collection of images that have been tagged or labeled with relevant information. This annotation can include identifying objects within the images, classifying images into categories, or providing critical contextual information. The goal of this process is to prepare these images for machine learning algorithms, allowing them to learn from the data and make predictions or classifications accurately.
Types of Image Annotations
There are several types of annotations that can be applied to images, which include:
- Bounding Box: A rectangular box drawn around an object in the image to identify its location.
- Semantic Segmentation: Each pixel in the image is labeled to denote different classes, providing a higher level of detail.
- Instance Segmentation: In this method, individual objects in an image are labeled separately, allowing for differentiation between instances of the same class.
- Landmark Annotation: Specific points are marked on an object for facial recognition and pose estimation applications.
- Text Annotation: Any text present in the image is labeled for applications like Optical Character Recognition (OCR).
The Role of Annotated Image Datasets in AI Development
Annotated image datasets serve as the critical training material for various AI applications. Without these datasets, machine learning models would struggle to understand and interpret the visual data they encounter. Here’s how they contribute to AI development:
1. Enhancing Model Accuracy
High-quality annotated image datasets significantly improve the accuracy and performance of machine learning models. The more precise the annotations, the better the model can learn to recognize patterns and features in new, unseen data. In fields such as medical imaging, where diagnosing diseases accurately is paramount, the role of detailed annotations is vital.
2. Accelerating the Development Process
Using established data annotation platforms, businesses can streamline their data processing workflows. Fast and efficient data labeling allows for quicker training and iteration, enabling teams to bring products to market faster. Platforms like KeyLabs.ai offer sophisticated annotation tools that integrate seamlessly into the AI development lifecycle.
3. Supporting Diverse AI Applications
Annotated image datasets are not just beneficial for a specific type of AI application. They support a wide range of use cases, including:
- Facial Recognition: Used in security systems, social media tagging, and user authentication.
- Autonomous Vehicles: Essential for object detection, lane detection, and interpreting traffic signals.
- Medical Imaging: Important for identifying tumors and other anomalies in radiological images.
- Augmented Reality: Facilitating the overlay of information in real-time through image recognition.
Benefits of Using Data Annotation Tools and Platforms
Investing in data annotation tools and platforms can provide numerous advantages for businesses looking to harness the power of AI through annotated image datasets. Here are several key benefits:
1. Improved Quality Control
Advanced data annotation tools incorporate features that ensure high-quality annotations. Automated quality checks, manual review processes, and AI-assisted labeling can significantly reduce errors and enhance the reliability of the datasets.
2. Scalability
As businesses grow and their data needs intensify, scaling up annotation processes becomes imperative. Data annotation platforms allow companies to handle large volumes of images efficiently, ensuring that they can meet the demands of their AI projects without compromising on quality.
3. Flexibility in Annotation Tasks
Different projects require different types of annotations. A robust data annotation platform provides versatility, allowing teams to switch between tasks like bounding box annotations, segmentation tasks, and more, all within a single interface.
4. Collaboration and Integration
Modern annotation platforms are built with collaboration in mind. Teams can work together in real time, share insights, and integrate with other tools such as machine learning frameworks, ensuring a smooth workflow from data gathering to model deployment.
Challenges in Annotating Image Datasets
While the advantages are substantial, there are challenges associated with creating high-quality annotations:
1. Time-Consuming Process
Annotating images can be labor-intensive and requires significant time investment, particularly for complex datasets. However, leveraging data annotation tools can mitigate this issue by automating parts of the process.
2. Subjectivity in Annotations
Annotations can sometimes be subjective, differing from one annotator to another. Establishing clear guidelines and standards is essential to minimize discrepancies and ensure consistency across the dataset.
3. Cost Implications
High-quality annotation services can be costly, particularly if outsourced. However, this investment is often justified by the improved model performance and faster time-to-market for AI products.
The Future of Annotated Image Datasets
The demand for annotated image datasets is only expected to grow as AI continues to permeate various industries. As technology progresses, several trends are emerging:
1. Automation in Annotation
AI-driven algorithms are becoming increasingly capable of performing initial annotation tasks. This automation can significantly reduce the time and effort required for human annotators, who can then focus on refining and verifying these annotations for accuracy.
2. Enhanced Quality Through AI
Machine learning models trained on existing datasets can assist in generating new annotations, leading to improved quality across datasets by identifying potential errors or suggesting better annotations based on learned patterns.
3. More Sophisticated Applications
As AI technology evolves, the scope of applications for annotated image datasets will broaden, encompassing advanced fields such as robotics, smart cities, and agriculture. This evolution will demand increasingly specialized datasets and annotation techniques.
Conclusion
In conclusion, annotated image datasets are integral to the success of AI and machine learning initiatives. As enterprises like KeyLabs.ai exemplify, the strategic use of data annotation tools and platforms greatly enhances the quality, efficiency, and scalability of datasets. Businesses that recognize the importance of investing in high-quality annotated datasets will position themselves advantageously in the competitive landscape of AI.
As we look toward the future, embracing innovations in data annotation will be crucial for leveraging the full potential of AI, transforming industries and enhancing the way we interact with technology.