Unlocking the Power of Machine Learning Data Labeling for Business Success
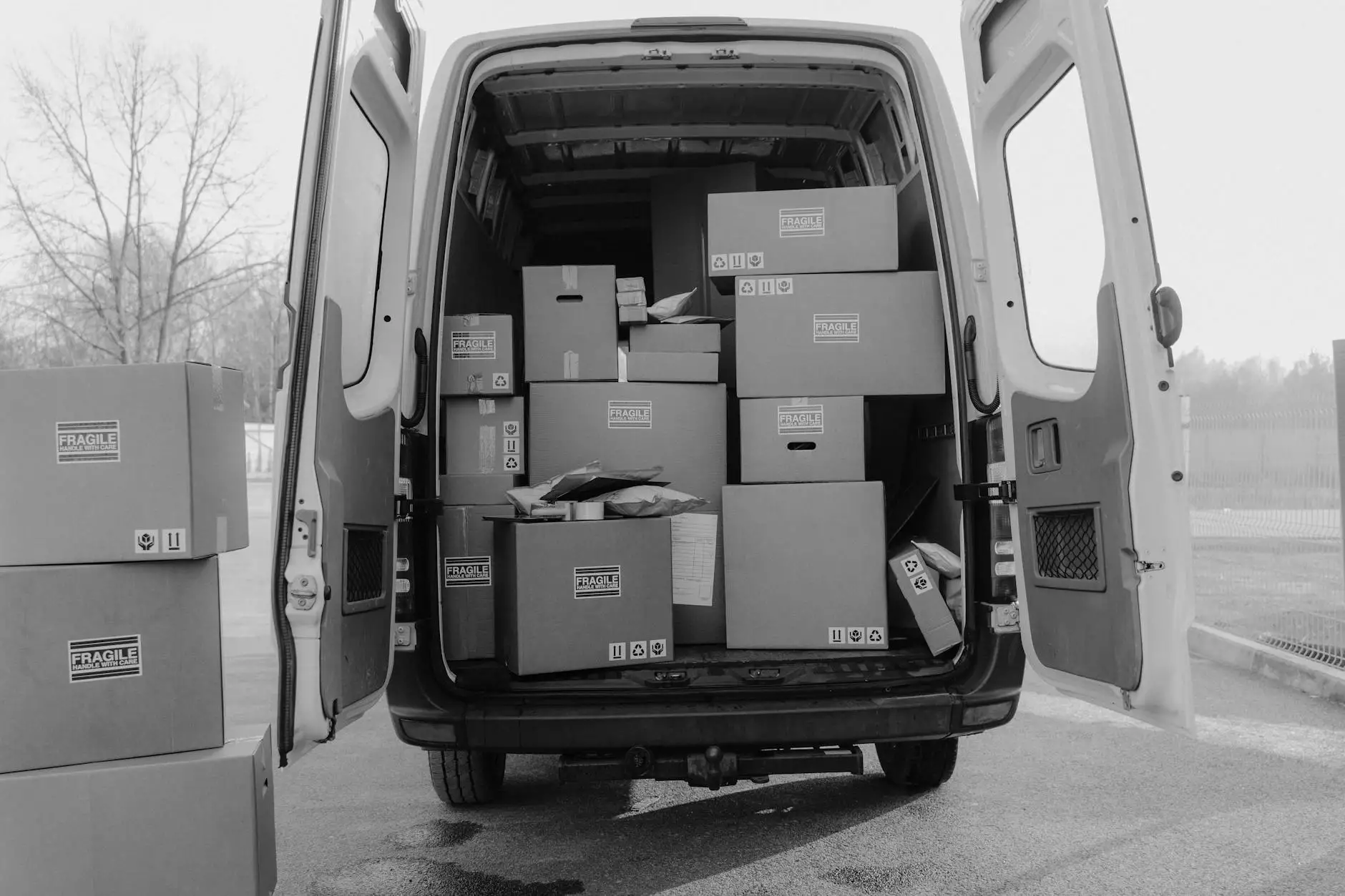
In today’s fast-paced business environment, technology is rapidly evolving, and one of the most significant advancements is in the field of artificial intelligence (AI) and machine learning. At the heart of making AI systems functional is the essential process of machine learning data labeling. This process is crucial for training algorithms, enhancing data quality, and ultimately driving informed business decisions. In this comprehensive article, we shall delve into the ins and outs of data annotation, explore its significance, and highlight how utilizing the right tools, such as those offered by KeyLabs.ai, can elevate your business performance.
Understanding Machine Learning Data Labeling
At its core, machine learning data labeling involves the meticulous process of tagging or annotating data to train machine learning models. This can include various types of data, such as:
- Images - Labeling objects or features in photographs to assist image recognition technology.
- Text - Annotating text for sentiment analysis, information extraction, or language processing.
- Audio - Transcribing and labeling sound recordings for voice recognition systems.
The Importance of Data Annotation in Machine Learning
Data annotation is vital for the success of any machine learning project. High-quality labeled data allows algorithms to learn patterns and make predictions effectively. Here are several key reasons why data labeling is significant:
1. Improved Model Accuracy
When data is accurately labeled, machine learning models can achieve higher levels of precision. This is essential for applications in various sectors, including:
- Healthcare - Accurate diagnostics through image and text analysis.
- Finance - Fraud detection mechanisms identifying anomalies in transaction data.
- Retail - Personalized marketing strategies driven by user behavior analysis.
2. Enhanced Data Quality
Quality labeled data leads to reliable outcomes. It mitigates risks associated with erroneous predictions that may stem from misunderstood data inputs. Leveraging high standards of data annotation helps businesses maintain integrity and trustworthiness in their AI systems.
3. Accelerated Product Development
With the expedited process of data labeling through automated tools or outsourcing, businesses can speed up their product development cycle. The quicker a model can learn from high-quality labeled data, the sooner a business can deploy its AI solutions, staying ahead of competition.
Why Choose KeyLabs.ai for Your Data Annotation Needs?
KeyLabs.ai stands as a notable solution provider in the field of machine learning data labeling. Here’s why businesses should consider their platform:
1. Cutting-Edge Data Annotation Tools
KeyLabs.ai offers powerful and versatile data annotation tools that cater to various machine learning projects. Their platform supports:
- Image Annotation - With features like bounding boxes and segmentation.
- Text Annotation - Ideal for NLP tasks including entity recognition and classification.
- Audio Annotation - Comprehensive tools for speech-to-text and sound classification.
2. Scalability
Whether you’re a startup or an established enterprise, KeyLabs.ai scales with your needs. Their infrastructure allows for handling large datasets efficiently, giving you the flexibility to expand your projects without any setback.
3. Human Expertise Combined with AI
While automation is a cornerstone of data annotation, human oversight remains indispensable. KeyLabs.ai blends cutting-edge technology with a team of experts who ensure the accuracy and quality of labeled data. This dual approach enhances the overall performance of machine learning algorithms.
Implementing a Successful Data Labeling Strategy
To gain optimal results from machine learning data labeling, businesses should implement a coherent strategy that encompasses a few key steps:
1. Define Objectives Clearly
Understanding what you want to achieve with data annotation will guide the labeling process. Objectives may include improving model accuracy for specific tasks or enhancing customer insights through data-driven analytics.
2. Select Relevant Data
Choose data that is pertinent to your objectives. Typically, the more diverse and comprehensive your dataset, the better the machine learning models will perform.
3. Decide on Annotation Methods
Whether to use automated annotation, manual labeling, or a hybrid approach should be dictated by your project's specifics. Each method has its benefits, and weighing them against project needs is crucial.
4. Quality Assurance
Establishing a robust quality assurance process is key. This involves regular checks and balances to ensure data is correctly labeled and meets the required standards.
Real-World Applications of Machine Learning Data Labeling
The application of machine learning data labeling spans various industries, showcasing its versatility and importance:
1. Automotive Industry: Self-Driving Cars
In the automotive sector, data labeling plays a crucial role in the development of self-driving technology. Cars require extensive datasets containing labeled images of road signs, pedestrians, and other vehicles for the algorithms to learn how to navigate in real time.
2. Retail: Enhanced Customer Experience
Retailers leverage customer behavior data annotated through machine learning to personalize shopping experiences and recommend products, consequently increasing sales and customer loyalty.
3. Healthcare: Diagnostic Insights
In healthcare, data labeling is a key element in developing models that analyze medical images or patient records, significantly enhancing diagnostic accuracy and disease prediction.
Future Trends in Machine Learning Data Labeling
As machine learning continues to evolve, several trends in data labeling are emerging:
1. Increased Automation
Continued advances in AI will lead to even greater automation in data labeling, reducing time and cost while maintaining high quality. Automated tools will become more sophisticated, streamlining the annotation process.
2. Collaborative Annotation Platforms
The rise of collaborative data annotation platforms will allow teams to work together efficiently. This strategy promotes knowledge sharing and faster turnaround times for labeled datasets.
3. Ethical AI Practices
As societal focus shifts toward ethical AI, data labeling will need to adhere to strict guidelines that ensure fairness and representativeness in the labeling process, minimizing biases in machine learning models.
Conclusion: The Strategic Necessity of Machine Learning Data Labeling
In a world driven by data, machine learning data labeling is not merely an accessory but a strategic necessity. By investing in quality data annotation practices through platforms like KeyLabs.ai, businesses can unlock the full potential of their AI initiatives, drive innovation, and gain a competitive edge. As technology progresses, embracing the right tools and methodologies in data labeling will undoubtedly pave the way for future growth and success.
Let KeyLabs.ai be your partner in navigating the complex landscape of machine learning data labeling. With our advanced tools, expert insights, and commitment to quality, your business can thrive in this data-centric era.